Optimal Dispatch Solution for Power Grids Integrated With Renewable Energy Sources
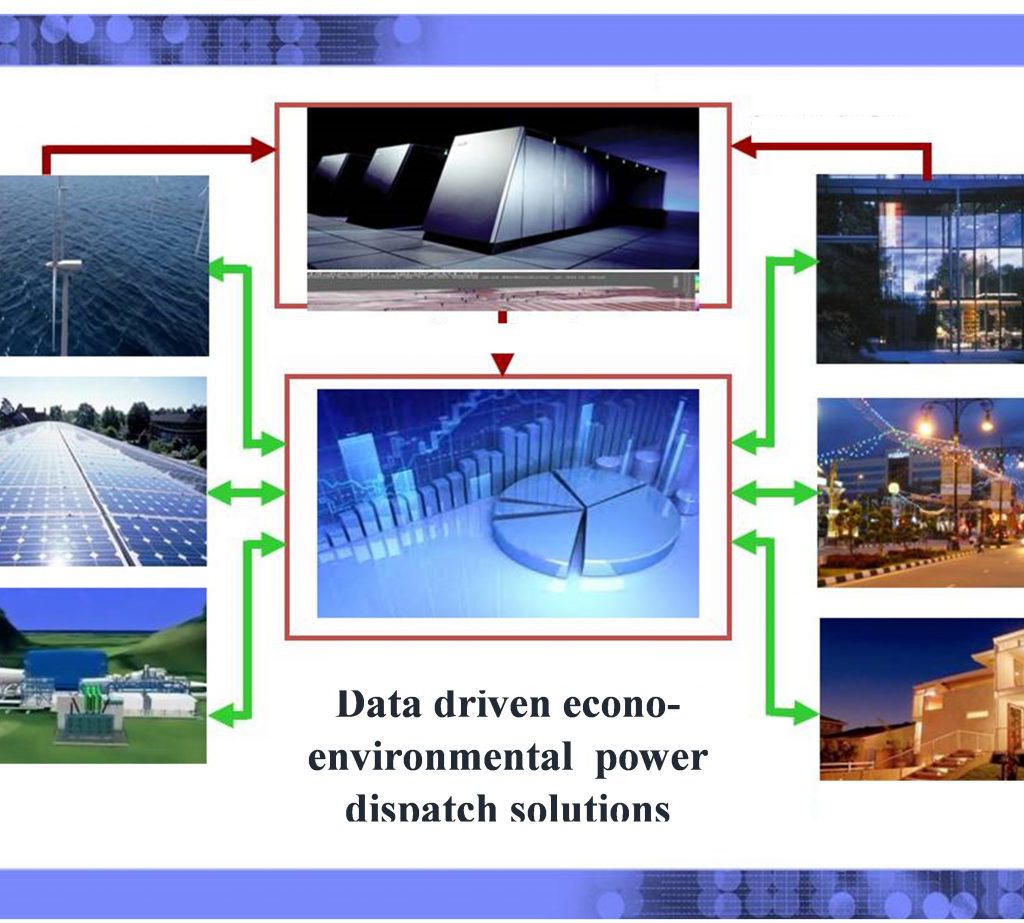
Figure 1: Proposed system for Econo-Environmental Dispatch (SEED).
Under this project, we are developing an Econo-Environmental dispatch system for power grids with substantial renewable penetration. The proposed system predicts the fluctuations in the demand as well as generation through machine learning. The grid system is also modeled for its capabilities and constraints. Then the generation is optimally matched with the demand for minimum cost of energy as well as the lowest level of emissions.
The temporal variations in the output from potential renewable sources like solar and wind are considered in the generation side. Ramping behavior of these renewable systems are modeled based on the long term performance data using Artificial Intelligence techniques. These models can give an insight to the contributions that can be expected from renewable generation in different time scales. To predict the load in a given time, a load forecasting system based on the novel “Environmental Sensitivity Index (ESI) approach has been developed. Once the load and generation requirements are quantified, the optimal dispatch solution could be suggested by the optimization engine.
Demand side management of energy consumption in the domestic sectors in Brunei Darussalam
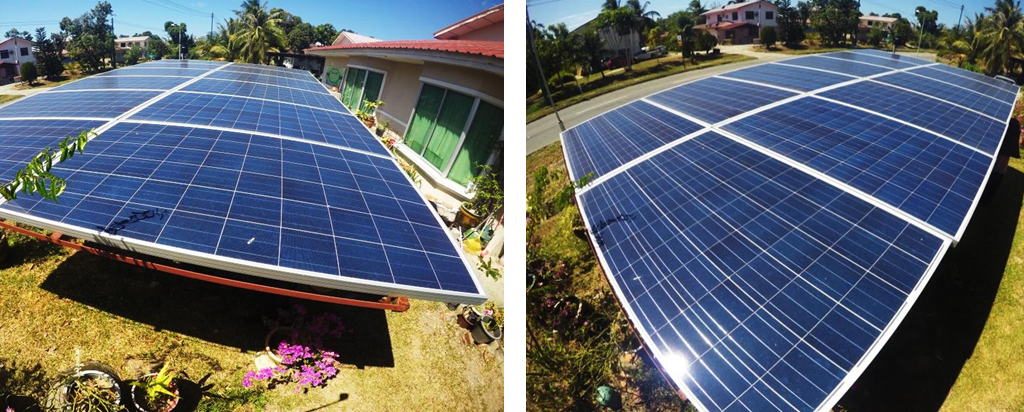
Figure 2: Solar PV systems installed in a house.
Brunei Darussalam is targeting to reduce 63% of the National energy consumption by 2035. Besides, at least 10% of the power generated would be from New and Renewables sources by this target year. Hence, energy efficiency and renewable energy use plays key roles in Brunei’s long term energy scenario. Household demand accounts for 38.2% of the total energy consumed in Brunei. Hence, the domestic sector should be a prime target for energy conservation and renewable energy utilization initiatives.
With these in mind, this project aims at identifying efficient demand side management of energy use in Bruneian homes. Long term energy demand in Brunei has been modelled using the LEAP. Energy demand for various sectors, under this 63% targeted reduction, has been modelled. Possible energy conservation measures in the household activities are identified based on the data generated from the previous pilot demonstration project carried out by the UBD|IBM Centre. Field level experiments are also conducted.
With an average daily solar insolation of 5.43 kWh/m2/day, solar energy is the most viable renewables energy for Brunei. Possibilities of roof top solar PV systems in Bruneian homes are also being investigated. Along with the technical aspects, a detailed cost benefit analysis following the present worth approach has also been carried out.
Short term wind power forecasting system combining Physical models with artificial intelligence techniques
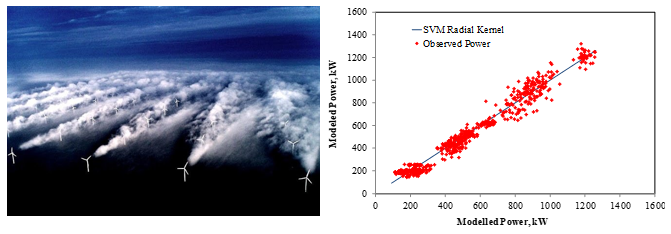
Wind being a stochastic phenomenon, the power output from wind energy systems may significantly vary from time to time, in tune with the temporal changes in the strength and direction of available wind resource. These frequent changes in output may pose significant challenges in grid integration of wind farms as well as the efficient dispatch of generated wind power. Hence, reliable forecasts of the power output from the farms, at different time intervals, are essential for the efficient management of wind energy projects.
Keeping in this view, a reliable wind farm power model has been developed employing artificial intelligence techniques. Models based on different machine learning methods are developed and their respective accuracies are estimated. These models can be trained with historic data on power output from a wind farm at a given wind speed, direction and stratification. A representative result, showing the performance of Support Vector Machines in forecasting the power output from an offshore wind farm, is shown in the figure. These models can further be integrated with the Numerical Weather Prediction (NWP) systems to forecast the wind farm output at different time scales.